主要是看想做一下利用深度学习进行图像重建的文献调研。此处所说的主要包含CT、MRI等医疗图像重建,超分辨图像重建与冷冻电镜图像重建。
MRI
Deep ADMM-Net for Compressive Sensing MRI - NIPS Proceedings
(NIPS 2016)
把MRI重建的ADMM算法展开成网络,原来的ADMM中的scalar都成为可学习参数,除此之外,用来正则化图像u的滤波器W用卷积取代,卷积核可学习;以及soft-threshold函数也用分段线性函数逼近,其中的各控制点的大小也是要学习的。因为核磁的线性变换实际上是一个傅立叶变换,所以ADMM算法中的求解线性模型可以用傅立叶逆变换实现,使得算法速度不会太慢。
ADMM-Net: A Deep Learning Approach for Compressive Sensing MRI
(Submitted on 19 May 2017)
上一篇文章的改进。有三点:
- generalize the ADMM-Net to a more general network structure achieving higher MR image reconstruction quality.
这一点主要指对于CS-MRI模型用ADMM求解,因为松弛变量选择的不同,可以推导出两种不同的迭代方法,上一篇文章是第一种算法展开,这一篇文章是第二种算法展开。
- extend the network to reconstruct the complex-valued MR image which is more useful in clinical diagnosis
所有的filter依然是实值,所以卷积层都是实数filter,复数的输入和输出。只有在非线性变化层(分段线性函数层)与实数网络会有不同实现的方法,即把虚部看做实数使用相同的分段线性函数处理再转回虚数。
- extensively evaluate the ADMM-Nets with different widths and depths, and demonstrate the superiorities of the networks by more comparative experiment
CT
Deep Convolutional Neural Network for Inverse Problems in Imaging
(Submitted on 11 Nov 2016)
文章的思路很简单,就是把FBP得到的不好的图片用一个U-net进行修正。不是很理解作者的思路,提到了ADMM-Net等网络结构,但认为那样不好,所以选取了U-Net网络结构。作者均是CS方向的,这可能反映了计算机和数学方向的不同理念。
While we were inspired by the general form of the proximal update, (6), to apply a CNN to inverse problems of this form, our goal here is not to imitate iterative methods (e.g. by building a network that corresponds to an unrolled version of some iterative method), but rather to explore a state-of-the-art CNN architecture.
Solving ill-posed inverse problems using iterative deep neural networks
(Submitted on 13 Apr 2017 (v1), last revised 22 May 2017 (this version, v2))
思路是从一个一般的正则化模型\[\frac{1}{2}\vert\vert f-Pu \vert\vert_2^2+\kappa\vert\vert \nabla u \vert\vert_2^2\]出发,使用梯度下降法会得到一个算法。我们参数化算法计算梯度的过程,“学习”一个梯度函数,按照这个梯度函数更新确定的步数,这就是Algorithm 1。另外,从Qusi-Newton类方法得到启发,如果利用上一步的信息,可以以更快的方向下降。所以引入了memory,从而得到Algorithm 2。
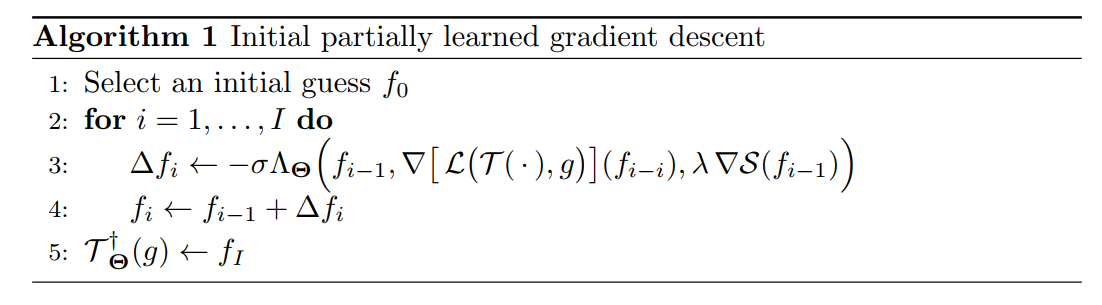
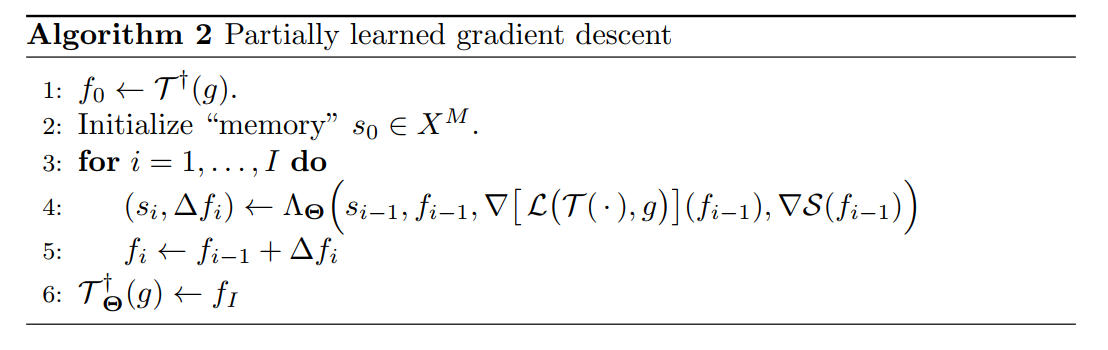
在数值实验中,网络结构是这样的:
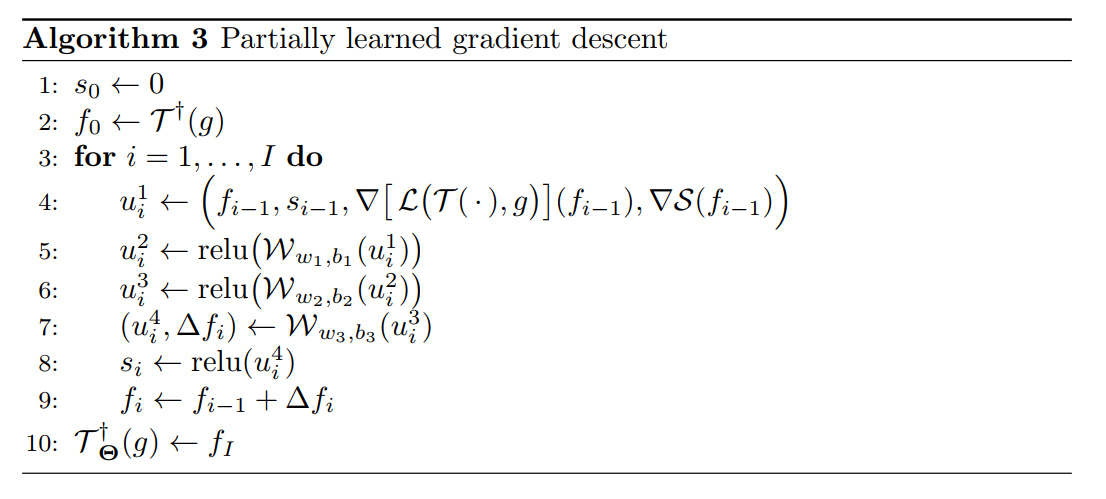
其中\[u_i^2\] ,\[u_i^3\] 都是32通道的,卷积核是\[3 \times 3\]的,\[I=10\],\[s_i\]是五通道的。
数据集有两种:
- Ellipses
图像为\[128 \times 128 \]大小,30views,5%噪声,对与原图的\[L^2\] Loss进行优化。
- Heads provided by Elekta (Elekta AB, Stockholm, Sweden)
图像为\[512 \times 512\] 大小,fan beam geometry with source-axis distance of 500 mm, source-detector distance 1000 mm, 1000 pixel, and 1000 angles.
采用了非线性的算子(我觉得与上一种的区别主要在于加噪声的方法不一样,所以线性算子使用\[L^2\]损失,而这个使用 K-L divergence)
\[\mathcal{T}(f)(l)=\lambda(-\mu \mathcal{P}(f)(l))\]
\[\mathcal{L}(\mathcal{T}(f),g):=\int_{\mathbb{M}}(\mathcal{T}(f)(l)+g(l)\log(\frac{g(l)}{\mathcal{T}(f)(l)}))dl\]